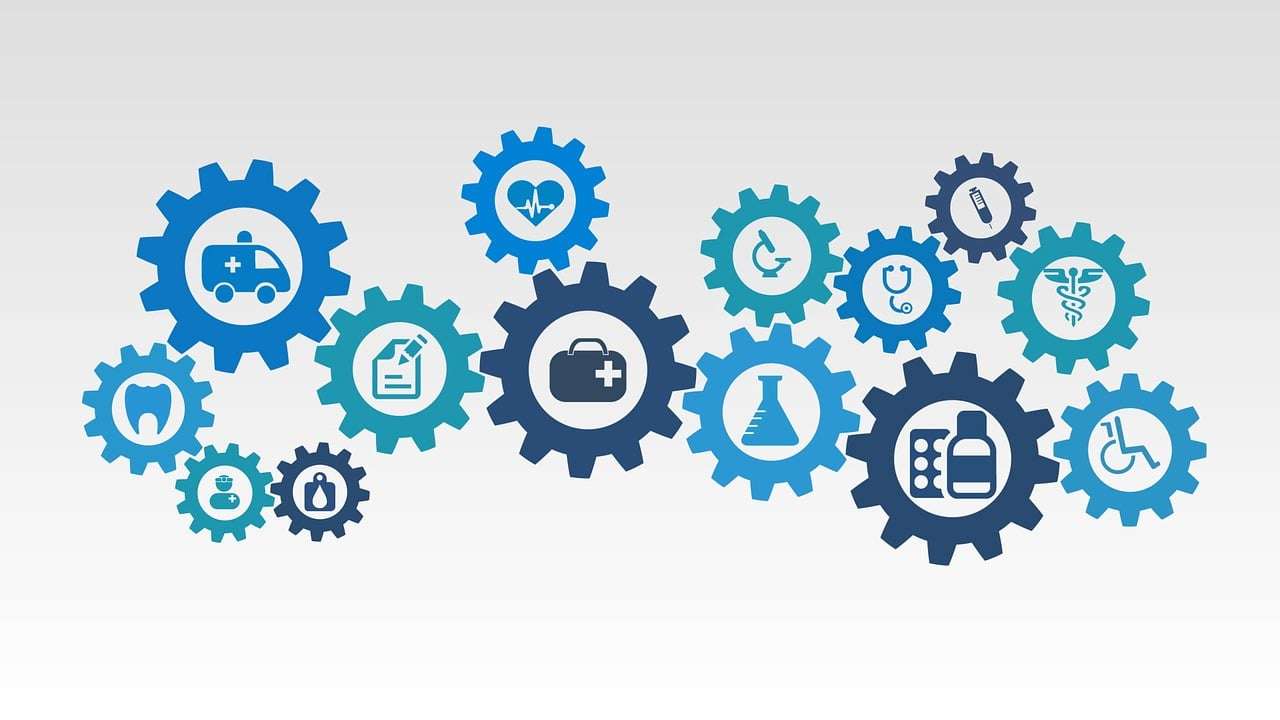
A health information technologies center connects people and systems at more than 27,500 contracted provider facilities worldwide. Recognized for innovation, the center offers solutions and services for health care organizations of every size. Together with its clients, it is creating a future where the health care system works to improve the well-being of individuals and communities.
“We are going in the direction of retiring OBIEE reporting. Our choice is to replace it with Power BI. Having data sets in azure makes it easier to start pivoting in that direction.”
Lead Technology Architect
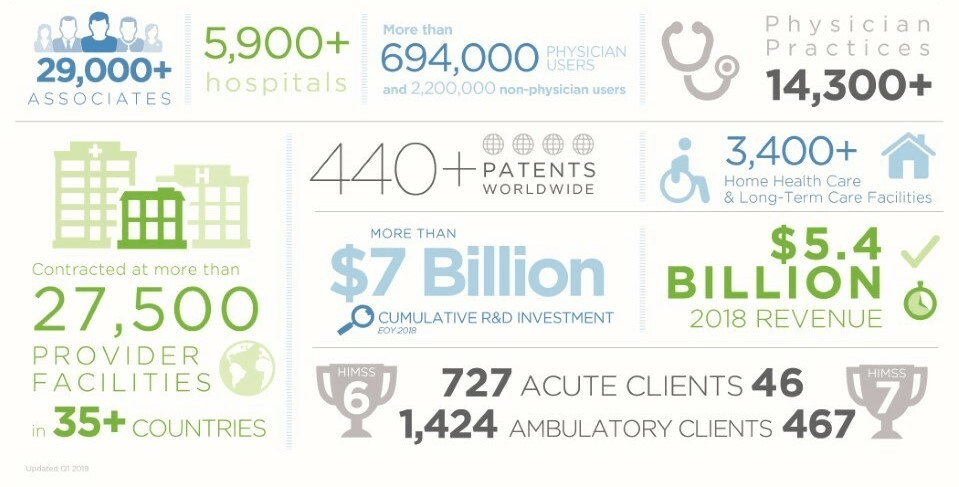
The Lead Technology Architect at Cerner Corporation recently helped the center develop a configuration management system in the cloud to give the company’s IT business operation teams one source of truth for its asset and configuration management inventory. Now, employees are better able to perform their jobs, spending time innovating and deploying code instead of simply maintaining assets.
The center’s disparate systems
Because the center is an IT company that delivers software to customers around the world, maintaining accurate, reliable, and current systems information is central to delivering value to customers. About three years ago, it was at a breaking point with the structure of its asset and configuration management. The company had multiple disparate sources of asset data that were managed by different teams, none of which maintained central accountability. Critical business operations like hardware asset management, capacity management, and vulnerability management didn’t have a single source of truth.
Ideally, the center’s employees should be able to pull data from the on-premises BMC Remedy system and trust that all information is correct. However, asset data was being stored in so many separate places, with no consolidated and consistent path into Remedy, that the data was often unreliable.
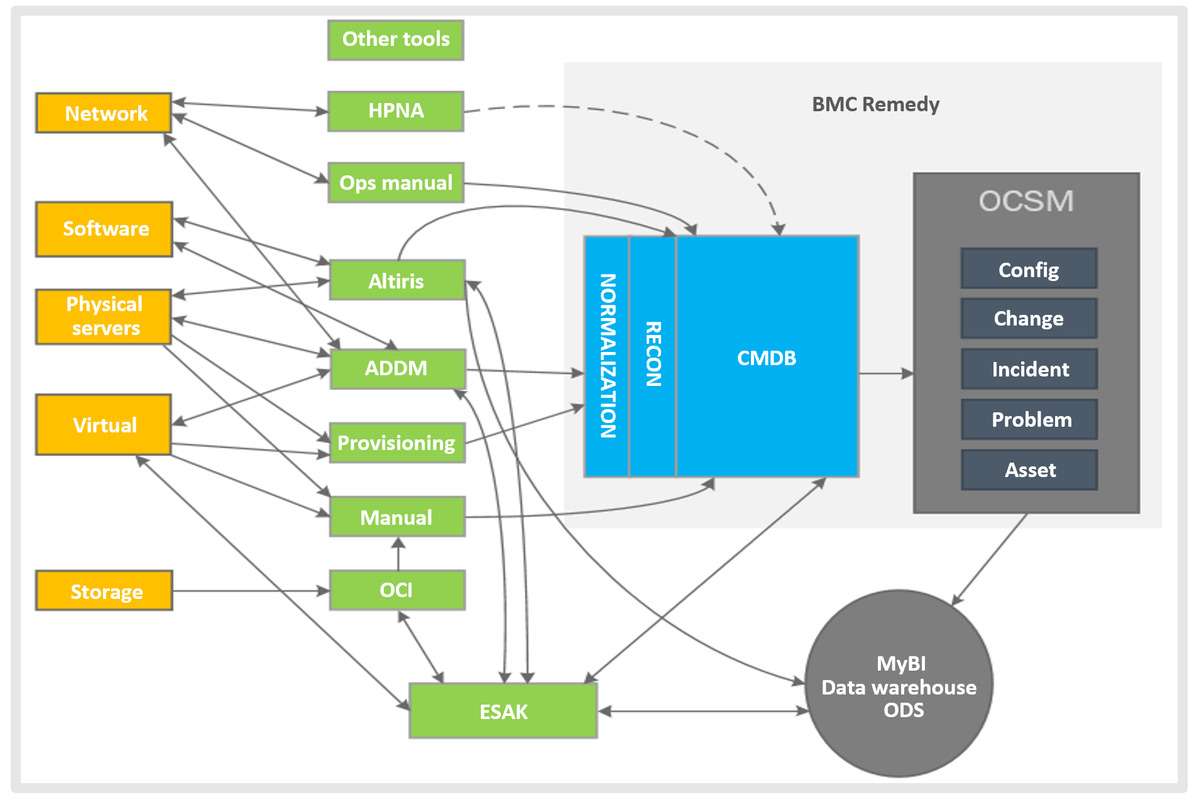
Whenever technical program managers needed information about company assets, they pulled data from one of several sources of asset data instead of directly using Remedy. The process of assembling data from the different sources and verifying its completeness would often take up to a week. After the dataset was complete, the technical program manager then required the respective team to validate that the dataset was complete and correct. Technical managers were wasting time collecting information on assets instead of focusing on tasks that push the business forward, such as developing and releasing code.
For example, a critical asset and configuration management task is being able to quickly and easily identify the number of servers in its environment. This seemingly simple calculation becomes complicated when the definition of a “server” changes depending on who you ask. Employees often presented different server counts based on their definitions of a server, and because of the center’s disparate sources of asset data, tracking where and how consumers arrived at a server count was nearly impossible. More time would then be spent trying to understand what servers were accounted for, making it difficult to know if upgrades and needed work had been completed on every required system.
A major part of the center’s core business process depends on consumers being confident that the data provided is of the highest quality. To check data quality, data is measured according to its data quality score. The score looks across five weighted dimensions: completeness, consistency, accuracy, validity, and conformity. Complex calculations were required to produce this score, which was calculated by employees manually combining data from multiple systems in Microsoft Excel spreadsheets. The process was long, tedious, and took an employee days to complete. Because of the intensity and manual labor required to produce this score, it was both updated for consumer use and presented to leadership only quarterly.
Another related problem was the time spent maintaining and cleaning the individual data sources. Each system performed differently in terms of aggregating, storing, and transferring data. Because each system has its own method of managing data, there was inconsistency in getting a holistic view of asset data.
Additionally, the center’s employees were using Oracle Business Intelligence Enterprise Edition (OBIEE) reports to visualize what was happening with assets in the company. The OBIEE reports recorded a snapshot of information at a specific time, and if a report was presented and further questions were asked, another report had to be created to find the answer, often from a different set of data. It needed reports with the ability to drill through information for a deeper interactive understanding of data, and the tool had to be able to hook into data sources on many different platforms.
Upper management was pushing for more cloud solutions at center for the low-cost and high-automation benefits. The center’s leaders wanted employees to spend time developing solutions, writing code, and innovating rather than performing tasks that could be done through the cloud. With its sights set on cloud solutions, it created a team to find solutions for the difficulties arising from the various methods of managing data.
Beacon is born
The lead technology architect was given responsibility to manage the creation of a new configuration management system that would maintain a reliable inventory of assets and configuration items, including the attributes and relationships necessary to support IT business and operations, and to automate as much of the process as possible. His mandate was to centralize data from all storage locations, allowing employees to access and analyze information more easily and to create one central source of data. The new system would establish a single source of truth that would result in clearer understanding of data, time saved on verifying and tracing data numbers, and increased data quality. The system was named Beacon.
Following upper management’s cloud strategy mandate, the lead technology architect and his team selected the Microsoft Azure data platform for its next-generation configuration management system. The team considered two main data storage technologies: Azure Blob storage and Azure Data Lake Storage. Although Blob storage had more integrations with technologies other than ADLS at the time, the decision was made to implement Data Lake Storage because of its enterprise-grade security, Active Directory Domain Services permission integrations, and the opportunities for analytics with various Azure services.
The next step was to figure out the best way to manage multiple data sources and workflows to get data in and out of the data lake. The Beacon team chose to use Azure Data Factory for the built-in connectors with most data sources and the UI for building workflows that don’t require writing code. To process the data out of the data lake, the center examined various enterprise data technologies and chose a variety of analytic tools, including Azure Data Lake Analytics and Azure Databricks because of the familiar SQL language and pay-per-job plans.
After the background Azure services were configured and started, Microsoft Power BI was the natural choice for visualization because it naturally integrates with Data Lake Storage, Azure SQL Database, and Apache Spark. While the connection points were simple and complete, Power BI also offered front-end features that were responsive and reactive to consumers diving deeper into Beacon data. Because of Power BI drill through capabilities, employees can now get answers to questions quickly without waiting for additional reports. Offers such as Q&A also make data consumption easier for less technical consumers.
The beacon solution architecture
The success of Beacon at the center has centered on Data Lake Storage, which is the big data storage technology that Beacon uses for aggregating and storing daily snapshots of asset and configuration data.
The Beacon team developed a solution for combining three types of data sources into the data lake. The first type of data source is on-premises. Where Data Factory connections aren’t yet supported, an open-source application named Rundeck is implemented to push on-premises data to the data lake. The second group of data is pushed directly from whatever source it’s into Data Lake Storage, while the third dataset is pulled into Data Lake Storage by using Data Factory. Through the wide variety of compute options that Data Lake Storage supports, large amounts of data that come from various sources are cleaned, enriched, and transformed in the data lake.
After retrieving data from Data Lake Storage, Data Lake Analytics and Databricks are used to aggregate and transform the data, and the updated data is stored back in the data lake or is loaded into SQL Database. Most consumers need to pull from similar sets of information. The additional storage location of the SQL Database instance provides consumers easier querying abilities with highly structured stored models that are convenient for most consumers.
When selecting a data visualization tool, the Beacon team discovered that Power BI was the only option that had a connector to allow consumers who also need to perform more complex queries with data directly from the data lake to do so. Therefore, Power BI is used when data is taken directly from Data Lake Storage, but also as the primary tool for consumers who are using data from SQL Database. Some employees still use Tableau, but Power BI is the primary tool because of its ease of connection to data sources and its advanced visualization capabilities.
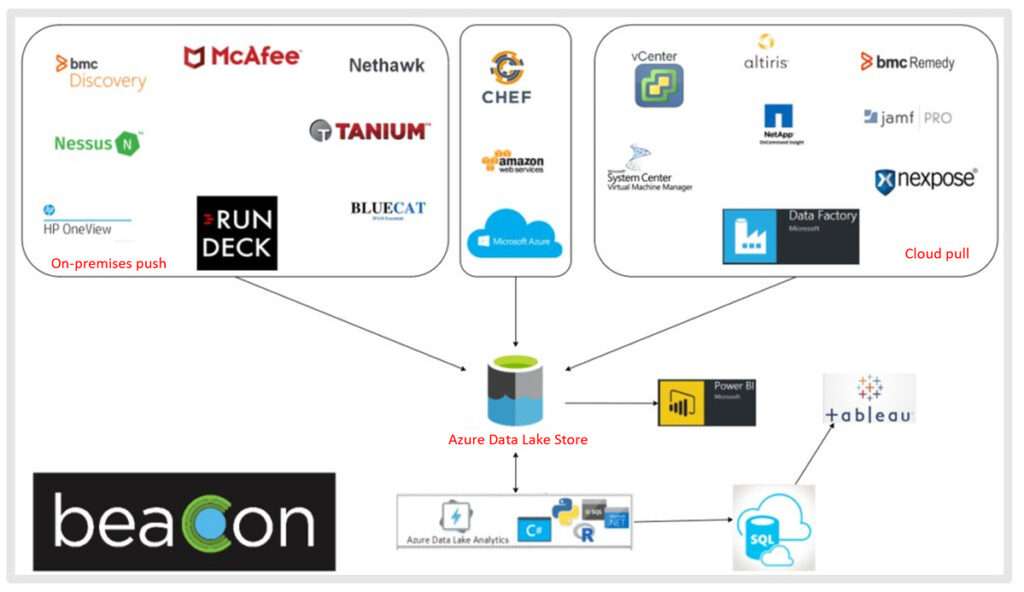
The benefits of beacon
As mentioned previously, one of the center’s asset and configuration management duties is determining how many servers are in the company’s environment in order to properly maintain each one and monitor the environment as a whole. Before Beacon, tracing where the data existed for a proper server count was complicated. Although its departments might still have different definitions of a server, the logic to get a final number is now easily traceable, eliminating the time consumed by proving where the numbers are pulled from. Beacon now uses Power BI to visualize its overall asset posture through a system count dashboard.
Because all data now lives in one place, the process of tracking the source of numbers is now much simpler. A user can drill through the count dashboard to see where the numbers come from. The centralized data location also makes it easier to analyze and compare what systems were counted and what systems were left out. Being able to understand the numbers and simplify the calculation became a reality because of the use of Azure and Power BI in Beacon. the center’s asset and configuration knowledge doesn’t end at producing server counts, though.
Prior to Beacon, the center couldn’t trust the quality of its data. With Beacon, the previously complex and manual calculation of its data quality score has now been simplified by using Data Lake Analytics and Databricks to create an automated process for quickly calculating these scores. Because all asset and configuration management data are now centralized in Data Lake Storage, the score can be calculated from data in one location rather than sifting through multiple sources. This one source of data eliminates data redundancy and increases the completeness of the data, creating more valuable and reliable scores.
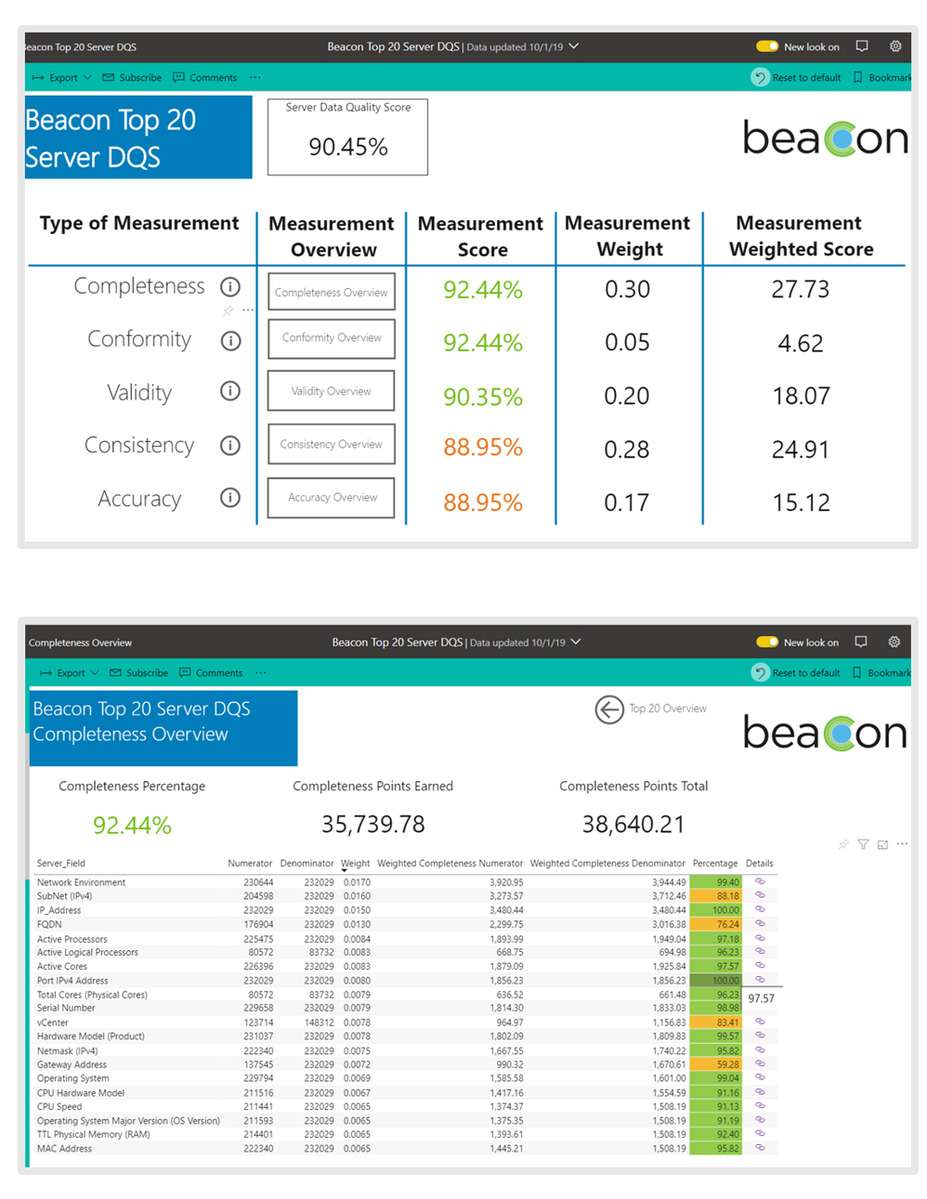
The automated process allows consumers to check on the quality of the data in the systems at any time. Consumers can continue drilling down into each field to examine every individual data point and whether it passed or failed a data quality check. This provides both consumers and Cerner’s development team transparency into gaps and what opportunities exist to improve the data.
Where gathering data and calculating a data quality score used to take days of manual work, the new process automatically provides an efficient and accurate calculation. Data quality scores that used to be available only quarterly are now available nearly on demand. Top-level management can now check data quality score calculations at the click of a button. Consumers can drill down into the calculations to make smart decisions on where to focus their time and energy to increase the quality of data.
The current status of beacon
Beacon has transformed the center’s configuration and asset management and succeeded in building a solution to create one source of truth for its data. After spending months gathering asset data from over 20 sources, it now has a single authoritative source of asset and configuration data that all consumers consistently use for security, operations, and financial use cases. The center is using Data Lake Analytics and Databricks to implement logic to analyze its disparate sources of data to determine what unique assets are active in its environment.
The Beacon solution was built almost entirely on Azure services, which has allowed the Beacon team to take advantage of tools that are designed to work together. And when issues occur, the team has found value in working with a single vendor to help diagnose where in the pipeline problems are originating instead of trying to work individually with multiple companies. The Beacon team also provides developers opportunities to innovate, brainstorm new ideas, and produce solutions instead of just maintaining them.
Next step for beacon
To extend the interactivity that Power BI Q&A provides, Beacon is piloting the use of Azure Bot Service. Consumers often have difficulty recognizing Beacon as a solution because it’s largely data—there’s no UI nor is there a URL that they can use to access Beacon like they do with most of the center’s other corporate services. Without a formal interface, consumers might become intimidated and worry that they don’t have the skills to analyze data for answers to their questions. The center is investigating presenting a guided, conversational interface where users can ask questions about data in natural language and receive an answer. The current proof of concept depicted in the following figure indicates that this vision is attainable.